In the fast-paced world of finance, making data-driven decisions is crucial. AI-driven financial analytics has revolutionized the way organizations handle investments, manage risks, and optimize operations. By leveraging artificial intelligence, businesses can uncover insights from massive datasets, predict market trends, and enhance financial decision-making with unparalleled accuracy.
This blog explores the role of AI in financial analytics, its key benefits, tools, and real-world applications. Whether you’re an individual investor or a financial institution, AI-driven analytics can transform the way you approach investments and financial management.
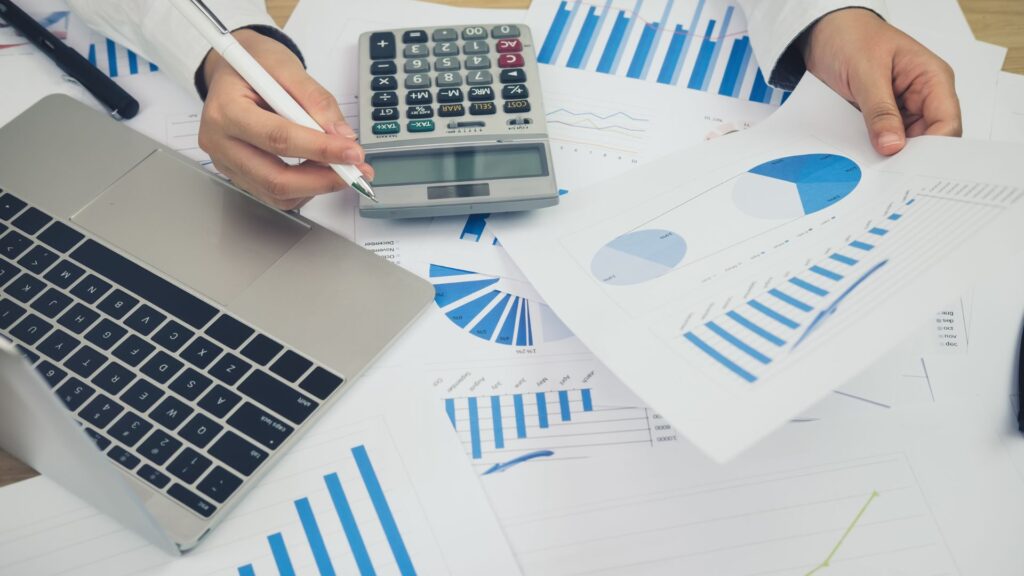
Why AI-Driven Financial Analytics is Essential for Investment Decisions
Financial analytics involves collecting, processing, and interpreting financial data to guide decision-making. Traditional methods often struggle to handle the volume and complexity of modern financial datasets. That’s where AI-driven financial analytics comes in, offering advanced tools to analyze data efficiently and extract actionable insights.
Enhancing Decision-Making with AI in Finance
AI models process vast amounts of data in real-time, identifying trends and correlations that human analysts might miss. By doing so, AI enhances decision-making, enabling businesses to respond quickly to market changes.
Predicting Market Trends with AI Analytics
Machine learning algorithms predict market trends by analyzing historical data, macroeconomic indicators, and real-time market fluctuations. This allows investors to anticipate opportunities and mitigate risks effectively.
Example Application:
An investment firm used AI analytics to predict stock price movements, achieving a 30% improvement in portfolio performance.
Key Benefits of AI-Driven Financial Analytics
The integration of AI in financial analytics offers numerous advantages, from enhanced accuracy to improved efficiency.
1. Improved Accuracy
AI-powered tools minimize errors by automating complex calculations and providing precise forecasts, ensuring more reliable results.
2. Faster Decision-Making
AI processes data much faster than traditional methods, enabling timely decisions that are critical in volatile financial markets.
3. Risk Management
AI models assess potential risks by analyzing financial data, geopolitical factors, and historical trends, helping businesses mitigate uncertainties.
4. Personalized Investment Strategies
AI-driven platforms create tailored investment strategies by analyzing individual investor profiles and preferences.
Internal Link: Explore our AI financial services.
External Link: How AI is revolutionizing finance – Forbes.
AI Tools for Financial Analytics
AI tools play a pivotal role in transforming financial data into actionable insights. Here are some of the most effective tools for AI-driven financial analytics:
1. Machine Learning Platforms
Platforms like TensorFlow and Scikit-learn enable the development of machine learning models for predictive analytics and market forecasting.
2. Natural Language Processing (NLP) Tools
NLP tools analyze financial news, earning reports, and market sentiment to provide a comprehensive view of market dynamics.
3. Automated Trading Systems
AI-powered trading systems like QuantConnect execute trades based on predefined algorithms, optimizing returns while minimizing risks.
4. Fraud Detection Solutions
AI solutions such as SAS and IBM Watson detect anomalies in financial transactions, safeguarding businesses against fraud.
Internal Link: Discover AI tools tailored for finance.
External Link: Best AI tools for finance – TechRadar.
How to Implement AI-Driven Financial Analytics
Integrating AI into financial analytics requires strategic planning and the right resources. Here’s a step-by-step guide to ensure successful implementation:
1. Identify Key Objectives
Define specific goals, such as improving investment strategies, enhancing risk management, or automating reporting processes.
2. Choose the Right Tools
Select AI tools that align with your organization’s needs, focusing on scalability, accuracy, and ease of integration.
3. Invest in Data Quality
AI models rely on high-quality data. Ensure your datasets are clean, complete, and free of inconsistencies.
4. Train Your Team
Provide training to your financial analysts and IT staff to help them understand how to use AI tools effectively.
5. Monitor and Optimize
Regularly evaluate the performance of your AI models and make adjustments to improve accuracy and efficiency.
Example Application:
A financial services company implemented AI-driven analytics to automate credit scoring, reducing loan approval times by 50%.
Applications of AI-Driven Financial Analytics
AI-driven financial analytics has a wide range of applications that transform how businesses and investors operate.
1. Portfolio Optimization
AI analyzes market data and investor preferences to create optimized portfolios that maximize returns while minimizing risks.
2. Market Sentiment Analysis
AI tools assess market sentiment by analyzing news articles, social media posts, and earnings calls, providing insights into investor behavior.
3. Fraud Detection and Prevention
AI detects suspicious activities in real-time, preventing fraudulent transactions and safeguarding financial systems.
4. Regulatory Compliance
AI ensures compliance by automating reporting processes and monitoring transactions for adherence to regulations.
Internal Link: Learn more about AI in finance.
External Link: AI applications in finance – Gartner.
Future Trends in AI-Driven Financial Analytics
The future of financial analytics is being shaped by advancements in AI technologies, promising even greater capabilities and efficiencies.
1. Real-Time Analytics
AI-powered platforms are enabling real-time data processing, allowing businesses to make instant decisions based on the latest market information.
2. Explainable AI
As AI adoption grows, explainable AI ensures that financial institutions understand how AI models generate insights, increasing trust and accountability.
3. Integration with Blockchain
AI and blockchain technologies are being combined to enhance transparency and security in financial transactions.
4. Predictive Analytics for ESG Investing
AI-driven predictive models are being used to evaluate Environmental, Social, and Governance (ESG) factors, enabling sustainable investment strategies.
Internal Link: Discover future AI trends in finance.
External Link: The future of AI in financial analytics – McKinsey.
Challenges in Implementing AI-Driven Financial Analytics
While AI offers transformative benefits, its implementation comes with challenges that need to be addressed for successful adoption.
1. Data Security and Privacy
Financial data is highly sensitive. Organizations must ensure compliance with data protection regulations like GDPR.
2. High Initial Costs
Implementing AI solutions can be expensive. However, the long-term benefits often outweigh the upfront investment.
3. Resistance to Change
Employees may be reluctant to adopt AI tools. Training and clear communication are essential to overcome resistance.
Internal Link: Contact us for tailored AI solutions.
External Link: Addressing challenges in AI adoption – Harvard Business Review.
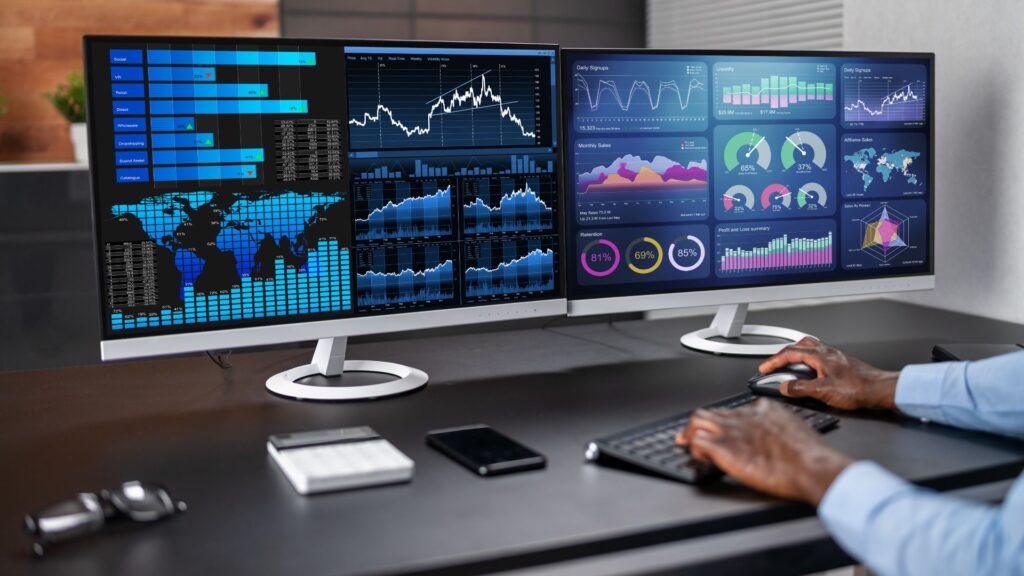
Conclusion
AI-driven financial analytics is transforming the financial industry, enabling businesses to make informed investment decisions, manage risks, and optimize operations. By leveraging AI-powered tools, organizations can unlock insights from data, enhance efficiency, and achieve their financial goals.
If your organization is ready to embrace AI-driven financial analytics, start by identifying key objectives, investing in the right tools, and building a skilled team. With a strategic approach, AI can revolutionize your financial decision-making and set you apart in a competitive market.